Upgrade 2021: phi LAB Speakers
September 21, 2021 // Upgrade 2021: PHI LAB Speakers
The Promise of Optical Neural Networks for Fast, Energy-efficient Computing
Peter McMahon, Assistant Professor of Applied and Engineering Physics | Cornell University
Summary
While currently computing is done only through electronics, technologies such as machine learning demand a faster, more energy efficient approach. In his talk at Upgrade 2021, Dr. Peter McMahon, assistant professor at the Cornell University School of Applied and Engineering Physics and director of the McMahon lab for research on physical computation, discussed the potential of using optics to perform neural network computations.
His goal is to demonstrate an optical neural network that uses tiny amounts of energy. Dr. McMahon explained that by using light to perform computations, there is the potential to use less energy in the system.
“The main motivation that we’re seeking is to build [neural networks] that are more energy efficient than what we currently have in digital CMOS hardware,” Dr. McMahon said, “There are some physical systems that can perform at least certain forms of computation far more quickly and energy efficiently than is possible to simulate with a digital computer.”
He specifically called out machine learning as an electronic computational system with room to improve energy efficiency through physical systems. Dr. McMahon explained the most costly aspect of machine learning is inference, or the process of running live data points into a machine learning algorithm. Typically, inference is responsible for around 80-90% of financial and energy cost.
“A company performing machine learning may have several engineers develop a machine learning model, but once they have trained it, they may then deploy it on a cloud-scale data center and have hundreds of thousands or millions or even billions of customers around the world use that model,” Dr. McMahon said, “The number of people doing the training versus the number of people doing inference with it can be vastly different.”
The physical properties of light fundamentally address key pitfalls in electronic computing, such as an increased amplifier bandwidth, larger spatial parallelism and dynamics that cost almost no energy at all.
Dr. McMahon explained the McMahon Lab’s researchers used a light source as the vector and amplify the optics through a spatial module before grouping the pixels and collapsing them down into single detectors. The results were astounding: optics as a physical computational system have the ability to use less than one photon of light per vector multiplication to achieve physical computing at 90% accuracy.
But researchers are still far off from computers that operate on optics alone. Data entry is one of the crucial aspects of machine learning that can currently only be done through electronics. Converting information from electronic systems to physical systems takes a significant amount of energy.
Regardless, the discoveries in the McMahon lab are promising. These findings prove that physical systems have the potential to reduce the both financial and energy costs when performing inference computation in machine learning.
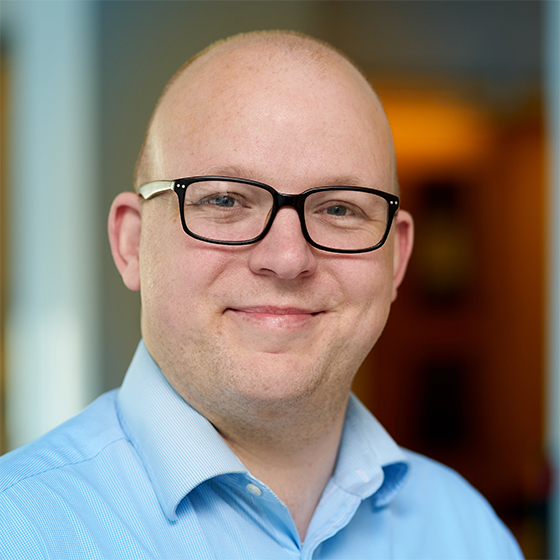
Peter McMahon
Assistant Professor of Applied and Engineering Physics | Cornell University
Peter McMahon received a Ph.D. in Electrical Engineering, with a Physics minor, from Stanford University in 2014, for research done on quantum information processing using optically controlled spins in semiconductors. He then performed postdoctoral work in the Department of Applied Physics at Stanford until he joined the faculty in the School of Applied and Engineering Physics at Cornell. Prior to attending graduate school at Stanford he spent one year at the University of California, Berkeley working on instrumentation for radio astronomy and bioinformatics. He received his undergraduate education at the University of Cape Town. While at Stanford he was awarded a Stanford Graduate Fellowship (2008 – 2011) and a Stanford Nano- and Quantum Science and Engineering Postdoctoral Fellowship (2015 – 2017).
MORE videos from NTT's upgrade summit, september 2021
- Satoshi Kako: CIM on Chip Demonstration
- Martin Fejer: Nonlinear Nanophotonics: Towards Few-Photons Interactions
- Ryan Hamerly: The Potential of Optical Neural Networks to Overcome Electronic Hardware Limitations
- Logan Wright: Physics-Aware Training for Deep Physical Neural Networks: From Digital Twins to Physics-Based Deep Learning
- Hiroki Takesue: Current Status of Coherent Ising machine/LASOLV at NTT Laboratories
- Hidenori Tanaka: Natural Science of Artificial Neural Networks
- Timothee Leleu: A Fast, Scalable, and Reconfigurable Simulation Platform for the Coherent Ising Machine