Upgrade 2020: PHI LAB Speakers
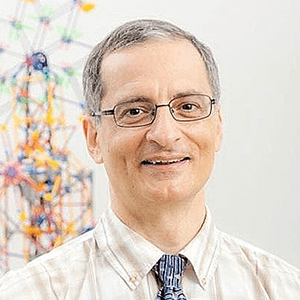
Franco Nori
University of Michigan
Machine Learning Applied to Computationally Difficult Problems in Quantum Physics
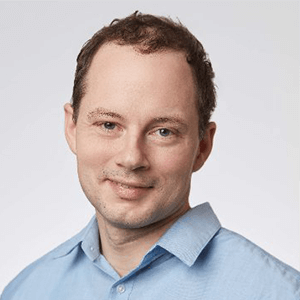
Dirk Englund
Associate Professor of Electrical Engineering and Computer Science at MIT
How Optical Technologies Overcome Limitations of Electronics in Machine Learning
Machine learning technology is facing limitations imposed by computational bottlenecks in electronics for tasks involving functions such as vision, games, control, and language processing. Dr. Dirk Englund, Associate Professor of Electrical Engineering and Computer Science at MIT, talked about the benefits of using photonic integrated circuits to overcome these bottlenecks.
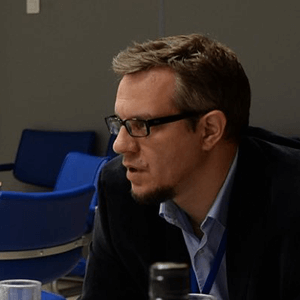
Zoltan Toroczkai
University of Notre Dame
The Path to Identifying Fundamental Limits of Continuous Time Analog Computing
Boolean Satisfiability (SAT), which was the first NP-complete problem to be identified in the 1970s, is a family of logical constraint satisfaction problems that are still intractable today. An efficient solution to this problem would translate to all other hard computing problems that are known to also be in NP, though the general consensus is that there is no efficient solution to such problems.
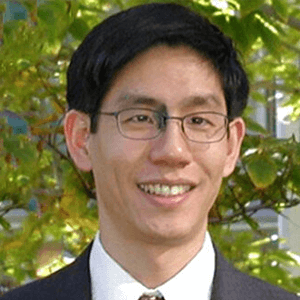
Isaac Chuang
Professor of Physics, MIT
New Thinking on Reducing Cost and Time in Programmable Quantum Simulators
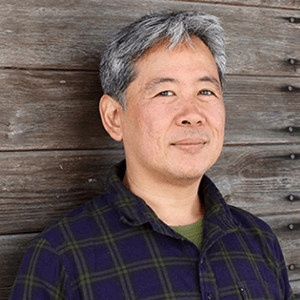
Hideo Mabuchi
Stanford University
How Coherent Ising Machines Can Bring Improvements to Combinatorial Optimization and other Computing Challenges
In his talk at Upgrade 2020, Dr. Hideo Mabuchi, Professor of Applied Physics at Stanford University…
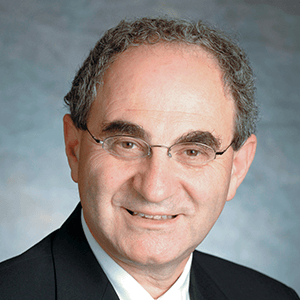
Eli Yablonovitch
Professor, UC Berkeley
Eli Yablonovitch Outlines the Promise of Physics-Based Optimization Principles for the Future of Computing
In the physical world we constantly witness examples of optimization under constraints. Light through a glass window takes the route that requires the least amount of time. A leaf falling from a forest canopy follows a path which generates the least entropy in its floating freefall. It is thus useful to recognize where optimization principles come into play, and exploit them to solve optimization problems across many fields, including electronic engineering.

Alireza Marandi
California Institute of Technology
Computing Opportunities Using Optical Parametric Oscillator Networks
As we reach limitations of standard computing, the need arises for different types of networks capable of solving incredibly complex and costly problems, from protein folding to social network optimization. In his talk, Dr. Alireza Marandi, Assistant Professor of Electrical Engineering and Applied Physics at Caltech, discussed the opportunities provided by Networks of Optical Parametric Oscillators (OPOs), which use the power of phase transitions for computation.
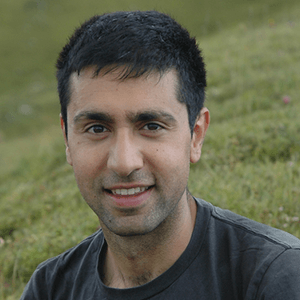
Amir Safavi-Naeini
Assistant Professor, Applied Physics, Stanford University
A Platform of Possibilities: Phononic and Photonic Circuits in Harmony
It’s possible to efficiently process both quantum and classical information on a single platform by employing mechanical motion and the efficiency of optics, according to Dr. Amir Safavi-Naeini, Assistant Professor of Applied Physics at Stanford University. In his Upgrade 2020 talk, Dr. Safavi-Naeini discussed his work-in-progress solution to overcoming limitations encountered with current platforms.
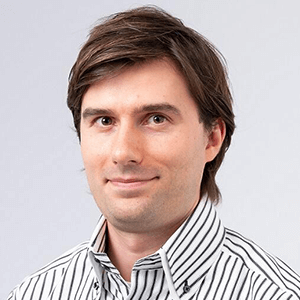
Timothee Leleu
University of Tokyo
Neuromorphic in Silico Simulator For the Coherent Ising Machine
The human brain is an inspiring model of what can be achieved computationally. To design a more effective simulation of a Coherent Ising Machine (CIM), we can use what we understand of the brain’s balance between speed, size, and energy efficiency.
“The brain computes using billions of neurons using only 20 Watts of power and operates at a relatively low frequency,” said Timothee Leleu, Project Professor of Information and Electronics at the University of Tokyo, in his talk at Update 2020.